Monitoring of Rice Growth with UAV-derived Aerial Imagery
doi: 10.14456/mijet.2019.4
Keywords:
Remote sensing, UAV, rice, biomass rice, vegetation indexAbstract
This study aims to monitor rice growth by using the reflectance relation of (R-B)/(R+B) and R/(R+G+B) to predict rice biomass before and after the heading stage. UAV-derived aerial imagery was obtained from an RGB camera attached on the UAV, which flew to take pictures at the altitude of 90 meters, with the front-overlap of 90% and side-overlap of 60%, to be used for calculating Green-Red Vegetation Index (GRVI) and Red Green Blue Index (RGBI). In addition, the field data were divided into two parts data for calibration and data for evaluation of three models through Rapid minder Studio 9.1, namely Generalized Linear Model, Deep Learning, and Random Forest. 120 sets of field biomass data were collected, 80 of which were for calibrating the models, and 40 for evaluating the models. After the evaluation of Coefficient of Determination (R2) and Root Mean Square Error (RMSE), for the biomass of rice before the heading stage, it was found that for GRVI, R2 and RMSE were 0.920 and 0961, respectively, and for RGBI, R2 and RMSE were 0.918 and 0.697, respectively. Meanwhile, for the biomass of rice after the heading stage, it was found that for GRVI, R2 and RMSE were 0.854 and 1.648, respectively, and for RGBI, R2 and RMSE were 0.810 and 1.530, respectively. For both periods, the most suitable prediction model was Random Forest. This shows that the reflectance relation of both equations based on GRVI and RGBI could be used to monitor rice growth.
References
[2] Niel, T.G.V. and McVicar, T.R. (2001). Remote Sensing of Rice-Based Irrigated Agriculture, December 15, 2009, from: https://www.clw.csiro.au/publications/consultancy/2001/CRC-Rice-TRP11050101.pdf.
[3] Shao, Y., Fan, X., Liu, H., Xiao, J., Ross, S., Brisco, B., Brown, R. and Staples, G. (2001). Rice monitoring and production estimation using multitemporal RADARSAT. Remote Sensing of Environment, 76, 310–325.
[4] Geipel, J., Link, J. & Claupein, W. 2014. Combined Spectral and Spatial Modeling of Corn Yield Based on Aerial Images and Crop Surface Models Acquired with an Unmanned Aircraft System. Remote Sensing 6(11): 10335-10355.
[5] Mulla, D.J. 2013. Twenty five years of remote sensing in precision agriculture: Key advances and remaining knowledge gaps. Biosystems Engineering 114(4): 358-371.
[6] Nuarsa, I.W. and Nishio, F. 2007. Relationships between rice growth parameters and remotesensing data. Journal of Remote Sensing and Earth Sciences 4: 102-112.
[7] Govender, M., Chetty, K. & Bulcock, H. (2007). A review of hyperspectral remote sensing and its application in vegetation and water resource studies. Water SA, 33(2), 145-151.
[8] Swain, K. C., & Zaman, Q. U. (2012). Rice crop monitoring with unmanned helicopter remote sensing images. Remote Sensing of Biomass-Principles and Applications. InTech.
[9] Kaewplang, S. & Srihanu, N. (2018). Remote Sensing with BNDVI and GRVI: Case Study of Field-Grown Rice in Khon Kaen, Thailand.
[10] Kawashima, S., and Nakatani, M. 1998. An algorithm for estimating chlorophyll content in leaves using a video camera, Ann. Bot. 81: 49-54.
[11] Cai, H., Haixin, C., Weitang, S., and G, Lihong, G. 2006. Preliminary study on photosynthetic pigment content and color feature of cucumber initial blooms, Trans. CSAE 22: 34–38.
[12] Hancock, D. W.; Dougherty, C. T. (2007). Relationships between blue- and red-based vegetation indices and leaf area and yield of alfalfa.
[13] Noureldin N.A., Aboelghar M.A., Saudy H.S., Ali A.M. Rice yield forecasting models using satellite imagery in Egypt. Egypt. J. Remote Sens. Space Sci. 2013;16:125–131.
[14] Motohka T., Nasahara K.N., Oguma H. and Tsuchida S. (2010). Applicability of Green-Red Vegetation Index for Remote Sensing of Vegetation Phenology. Remote Sens, 2, 2369-2387.
[15] Rahman A., Roytman L., Krakauer N.Y., Nizamuddin M., Goldberg M. Use of vegetation health data for estimation of Aus rice yield in Bangladesh. Sensors. 2009;9:2968–2975. [PMC free article] [PubMed]
[16] Huang, Y., Y. Lan, W. C. Hoffmann, and B. Fritz. (2008). Development of an unmanned aerial vehicle-based remote sensing system for site-specific management in precision
[17] Neti S.,Siwa K. (2018)Rice Yield Estimation from MODIS Data by Using Artificial Neural Networks Journal of the Association of Remote Survey Data and Geographic information of Thailand. 2018; Special Issue (19): 146-153.
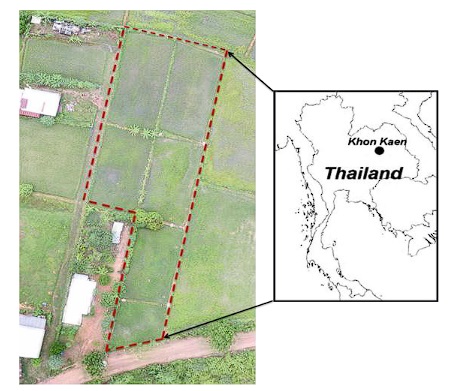
Downloads
Published
How to Cite
Issue
Section
License
Copyright (c) 2019 Mahasarakham International Journal of Engineering Technology

This work is licensed under a Creative Commons Attribution-NonCommercial-NoDerivatives 4.0 International License.